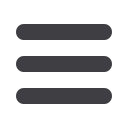
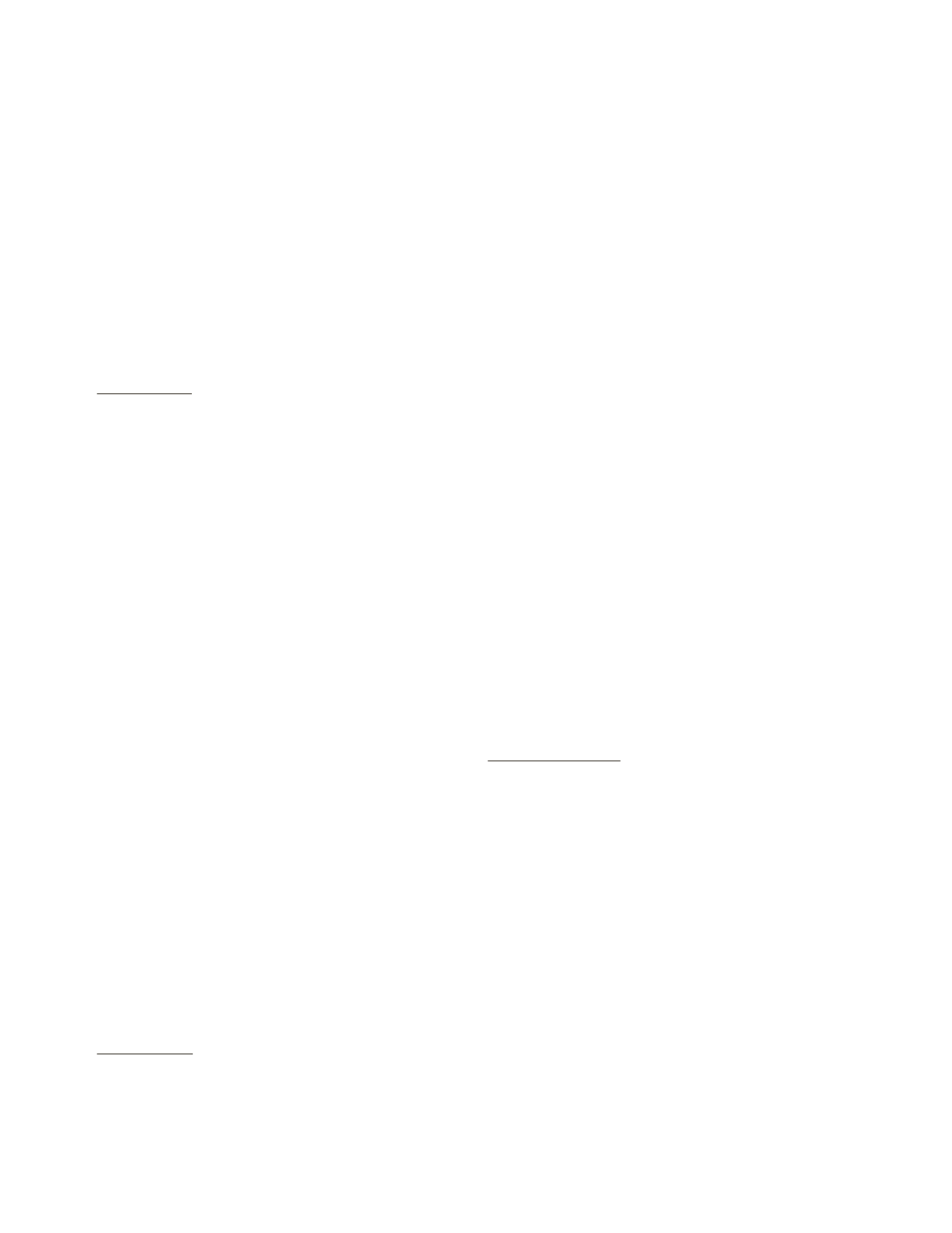
versus sham APP/PS1. Histological analysis at 48h revealed increased
6E10
+
ve A-beta deposits, microglial activation (Iba-1), argyrophilic
fibre (silver staining), and axonal bulb-like structures (phospho-
neurofilaments) in injured versus sham APP/PS1. Together, these
results support the hypothesis that CHIMERA TBI induces inflam-
mation, white matter pathology, and A-beta deposition in acute post-
injury period. Future studies will reveal how CHIMERA TBI affects
disease progression in APP/PS1 mice.
Keywords: Novel TBI Model, Mild TBI, APP/PS1 Mouse Model of
AD, Inflammation, White Matter Pathology
D2-10
DYNAMIC PROFILING: OUTCOME PREDICTION IN TBI
BASED ON DEMOGRAPHICS, INJURY CHARACTERISTICS,
AND INFLAMMATORY MEDIATORS
Andrew Abboud
, Gregory Constantine, Ava Puccio, Marius Buliga,
Alexey Solovyev, Qi Mi, David Okonkwo, Yoram Vodovotz
University of Pittsburgh, Surgery, Pittsburgh, USA
Objectives:
Inflammation induced by traumatic brain injury (TBI)
can lead to both morbidity and mortality. The goal of the present study
was to develop dynamic, data-driven computational models in order to
predict the likelihood of mortality post-TBI.
Methods:
Thirteen inflammatory cytokines and chemokines were
determined using Luminex in serial cerebrospinal fluid samples
from 31 TBI patients (26 survivors [24 males/2 females] and 5 non-
survivors [4 males/1 female]). Overall, patients in the cohort were 33
–
3
years old, with a mean Glasgow Coma Scale (GCS) score of 6
–
0.2.
Data on each subject, consisting of ten clinical (one-dimensional) var-
iables, such as age, gender, GCS score, Glasgow Outcome Scale
(GOS) score, and presence of infection, along with inflamma-
tory mediator time series were used to develop a technique called
‘‘Dynamic Profiling’’. Dynamic Profiling attempts to recreate the
clinician’s decision making process, by clustering patients sequen-
tially over time with regard to likelihood to die, using Hartigan’s
k-means method, into disjoint groups at different stages based on
demographic, injury, and inflammation data.
Results:
Using the Dynamic Profiling method, we could segregate
patients over time with regard to their mortality odds. This model had
a predictive accuracy of 72% for non-survivors.
Conclusions:
A novel, data-driven method was developed to assess
the probability of morbidity and mortality following TBI. This method
incorporates both injury-specific and demographic data as well as a broad
panel of inflammatory markers. Outcome prediction in the setting of TBI
may be improved by use of the Dynamic Profiling method, which in
essence replicates physician decision making in the setting of TBI.
Keywords: Dynamic Profiling
D2-11
DIFFERENTIAL DYNAMIC NETWORKS OF INFLAMMA-
TION IN CEREBROSPINAL FLUID OF TRAUMATIC BRAIN
INJURY SEGREGATE SURVIVORS & NON-SURVIVORS
Andrew Abboud
, Gregory Constantine, Ava Puccio, Qi Mi, David
Okonkwo, Yoram Vodovotz
University of Pittsburgh, Surgery, Pittsburgh, USA
Objectives:
Though inflammation induced by traumatic brain injury
(TBI) is a mediator of morbidity and mortality, its complexity has
defied therapeutics and diagnostic applications. We have previously
suggested that inference of dynamic inflammation networks may aid
in filling this gap. Thus, we hypothesized that differential dynamic
inflammation programs characterize TBI survivors vs. non-survivors.
Methods:
Thirteen inflammatory cytokines and chemokines were
determined using Luminex in serial cerebrospinal fluid (CSF)
samples from 31 TBI patients over 5 days. In this cohort, 5 were non-
survivors (Glasgow Outcome Scale [GOS] score
=
1) and 26 were
survivors (GOS
>
1). Significant differences in the time courses of CSF
inflammatory mediators were determined by Two-Way ANOVA.
Principal Component Analysis (PCA) was used to identify signatures
and key drivers of the inflammatory response. Dynamic Bayesian
Network (DyBN) inference was used to define central nodes of positive/
negative feedback, as well as defining outcome-specific dynamic in-
terrelationships among inflammatory mediators.
Results:
A Pearson correlation analysis of GCS vs. GOS suggested
that survivors and non-survivors had distinct clinical response tra-
jectories to injury. Statistically significant differences (p
<
0.05) in
interleukin (IL)-4, IL-5, IL-6, IL-8, IL-13, and tumor necrosis factor-
a
(TNF-
a
) were observed between TBI survivors vs. non-survivors by
Two-Way ANOVA. PCA suggested that IL-6 and IL-8 were hall-
marks of the post-TBI inflammatory response, whereas macrophage
inflammatory protein-1
a
(MIP-1
a
) and IL-10 were key component of
the inflammatory response in non-survivors. DyBN inference sug-
gested a core module of self-feedback and cross regulation among
IL-6, IL-8, and IL-1
a
in TBI survivors, with multiple mediators such
as TNF-
a
and IL-1
b
as output nodes. In contrast, DyBN suggested that
IL-6 and IL-8 alone were central nodes in TBI non-survivors.
Conclusions:
Differential dynamic trajectories and network pat-
terns elucidated by
in silico
modeling highlight the importance of IL-6
and IL-8 as principal drivers and central nodes in both survivors and
non-survivors following TBI, with a potential role for IL-1
a
in sur-
vivors.
Keywords: TBI
D2-12
DEVELOPMENT AND CHARACTERIZATION OF A ZEB-
RAFISH MODEL OF TBI
Victoria McCutcheon
1
, Eugene Park
3
, Elaine Liu
3
, Pooya
SobheBidari
4
, Jahan Tavakkoli
4
, Andrew Baker
2,3,1
1
University of Toronto, Institute of Medical Sciences, Toronto, Ca-
nada
2
University of Toronto, Anesthesia and Surgery, Toronto, Canada
3
St. Michael’s Hospital, Trauma Research, Toronto, Canada
4
Ryerson University, Physics, Toronto, Canada
Background:
Traumatic brain injury (TBI) is a leading cause of death
and morbidity in industrialized countries with considerable associated
direct and indirect healthcare costs. Animal models have been critical in
efforts to understand the pathophysiology of TBI, and to aid in the
identification of novel therapies. To date, numerous models of closed-
head trauma have been developed to address mTBI sequelae. However,
even with the use of rodent models, preclinical drug evaluation is a
lengthy process. In this regard, the zebrafish (ZF) has numerous advan-
tages to address the technical and time-dependent obstacles associated
with preclinical drug validation. There is a high degree of evolutionary
conservation between ZF and human homologue proteins, brain struc-
tures, and pathways. Furthermore, ZF offer advantages compared with
other vertebrate models, including the availability of rapid and efficient
tools for genetic manipulation and significant potential advantages in live
imaging and documentation of injury progression.
Methods:
We developed and characterized a ZF model of TBI
designed for high-throughput drug screening. In adult zebrafish, a
A-104